Bruno Loureiro
ENS & CNRS, Departement d'Informatique.
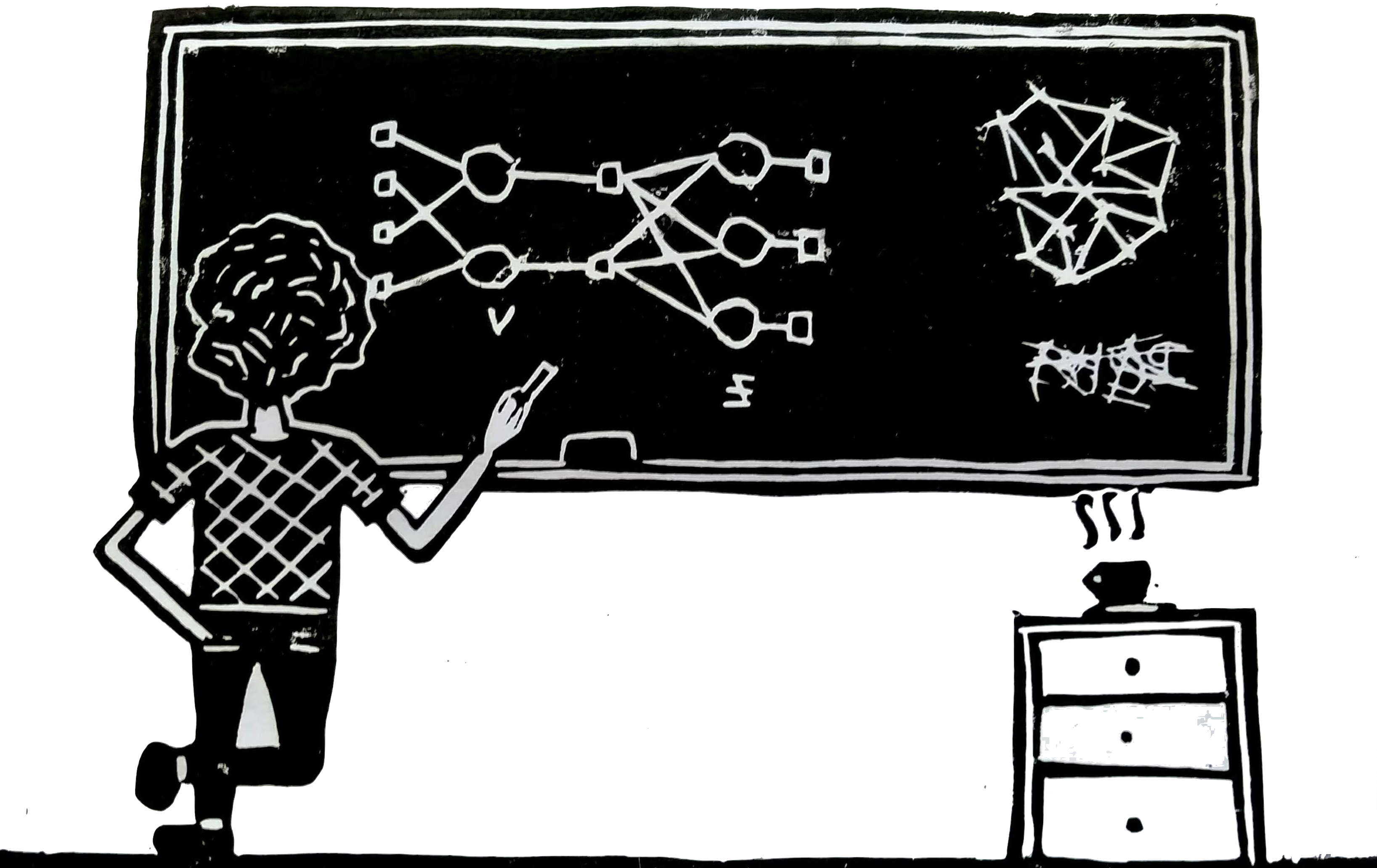
I am a CNRS researcher based at the Centre for Data Science at the École Normale Supérieure in Paris working on the crossroads between machine learning and statistical mechanics. I am also a Professeur Attaché at the Université Paris Sciences et Lettres (PSL) where I teach at the undergrad and graduate programs of the affiliated universities.
If you are interested in my research, you can find a complete list of my publications here.
news
Nov 03, 2024 | Our paper How two-layer neural networks learn, one (giant) step at a time was accepted for publication at JMLR! |
---|---|
Sep 26, 2024 | Our work Dimension-free deterministic equivalents for random feature regression has been accepted as a spotlight at NeurIPS 2024. Come see our poster in Vancouver! |
Sep 13, 2024 | I will be teaching a statistical physics of learning course at 15th edition of the Bangalore School on Statistical Physics, a traditional event organised by the International Centre for Theoretical Sciences (ICTS). Looking forward! |
Aug 01, 2024 | Excited to teach a course on statistical physics methods for machine learning theory Princeton Machine Learning Theory Summer School this summer. I have prepared some lecture notes, check them out if you are interested! |
May 02, 2024 | Our three works: were accepted for publication at ICML 2024! See you in Vienna in July! |